Guest Speaker Series: Opportunities in Machine Learning
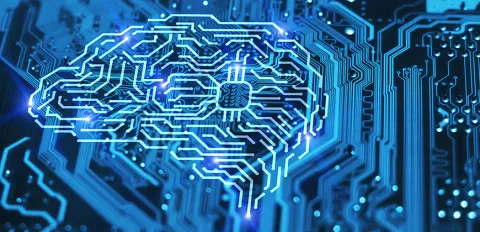
- Master of Engineering Leadership
On Wednesday, March 10, Amir Abdi hosted a seminar on the topic “Machine Learning: Challenges and Opportunities,” for UBC's Master of Engineering Leadership (MEL) students.
The presentation focused specifically on machine learning as a system, and the most pressing challenges of deploying and maintaining reliable and accessible machine learning services in production.
This seminar was particularly relevant for our MEL in Dependable Software Systems cohort, who were granted the opportunity to directly connect with and learning from a machine learning industry leader, and connect this new knowledge with their current classes on dependable software.
Leading innovation in dependable artificial intelligence
Amir Abdi is currently a Machine Learning Scientist/Engineer at Borealis AI, an RBC Institue for Research. Borealis AI is dedicated to achieving state-of-the-art in machine learning, with focus on ethical AI that will help communities thrive. Within his role, Abdi develops machine learnings models in the FinTech (financial technology) industry. Abdi has an extensive academic and professional history in both the theoretical and applied aspects of machine learning. He holds a PhD in Electrical & Computer Engineering - Machine Learning from UBC, and a Master's in Computer Engineering - Artificial Intelligence from the Sharif University of Technology. Further, Abdi is the co-founder and CTO of Offerland, a PropTech (real estate intelligence) startup in Vancouver.
Three Key Principles of Machine Learning
In his presentation, Abdi focused on exploring three key principles of machine learning, so that students had a more holistic understanding of the machine learning system.
- Failure Prevention
- Failure Identification & Reliablity Monitoring
- Model Maintenance
1. Failure Prevention – how can I prevent or reduce the likelihood of failure?
The most important thing to control for in failure prevention is the likelihood of bad data. Generally, bad data is a result of biases that permeated data collection. Data collection ought to be carried out in a wholly objective manner, by considering all sources of data and removing any possible errors or prejudices.
2. Failure Indentification & Reliability Monitoring – how can I identify failures and their causes when they occur?
Abdi commented on how, over time, sources of data will change and shift, and as a result, the machine learning model will no longer be accurate. For this reason, it’s incredibly important to actively monitor the data for shifts and raise any alerts before the accuracy of the model deteriorates.
3. Model Maintenance – how can I fix or address failures when they occur?
Model maintenance is based on ensuring that all strategies or plans are implemented to update and retrain the machine learning model. Over time, machine learning models may become broken through general deterioration. Abdi states that even the best models cannot capture all the changes that occur during production. The model must be maintained in light of all changes, in order to ensure that the results the model produces are trust-worthy and can be used in decision-making.
Creating ethical and reliable machine learning models
MEL students who attended this session learned the importance of machine learning, and the myriad of opportunities it creates. Abdi argued that desired behaviour cannot be effectively implemented in software logic without dependency on external data, creating the need for the use of machine learning that can be trusted.
MEL students in Dependable Software Systems in particular, gained industry knowledge on the importance of dependable AI and were inspired to lead positive change in the industry.
Are you passionate about creating reliable and ethical software systems? Explore the Master of Engineering Leadership in Dependable Software Systems to learn more about how this professional graduate degree can advance your career.